Big Data and Business Intelligence: Transforming Data into Strategic Insights
In today’s data-driven business landscape, the convergence of Big Data and Business Intelligence (BI) has revolutionized how organizations harness and leverage data to gain actionable insights and drive strategic decision-making. Here’s how the integration of Big Data and BI is transforming the way businesses operate:
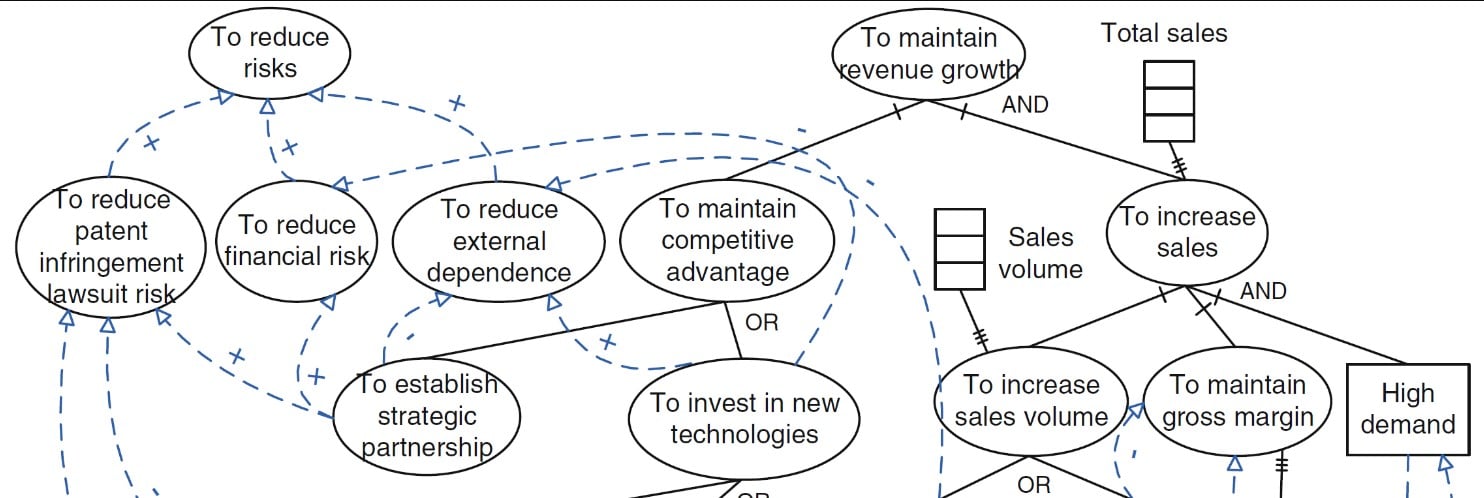
1. Descriptive Analytics:
Definition: Descriptive analytics focuses on summarizing historical data to understand what has happened in the past.
Purpose: Descriptive analytics provides insights into past performance, trends, and patterns, enabling organizations to identify areas of strength and weakness.
Examples: Key performance indicators (KPIs), trend analysis, data visualization (e.g., charts, graphs, dashboards).
2. Diagnostic Analytics:
Definition: Diagnostic analytics investigates why certain events occurred by analyzing historical data and identifying root causes of problems or trends.
Purpose: Diagnostic analytics helps organizations understand the factors driving specific outcomes and enables them to diagnose problems and make improvements.
Examples: Root cause analysis, correlation analysis, comparative analysis.
3. Predictive Analytics:
Definition: Predictive analytics uses historical data and statistical algorithms to forecast future trends, outcomes, and behavior.
Purpose: Predictive analytics enables organizations to anticipate future events, identify potential risks and opportunities, and make proactive decisions.
Examples: Regression analysis, time series forecasting, machine learning models (e.g., predictive algorithms, decision trees).
4. Prescriptive Analytics:
Definition: Prescriptive analytics recommends actions or strategies to optimize outcomes based on predictive models and business rules.
Purpose: Prescriptive analytics helps organizations make data-driven decisions by providing recommendations on the best course of action to achieve desired objectives.
Examples: Optimization algorithms, decision support systems, simulation models.
5. Cognitive Analytics:
Definition: Cognitive analytics combines artificial intelligence (AI) and advanced analytics techniques to mimic human thought processes and make sense of complex data.
Purpose: Cognitive analytics helps organizations extract insights from unstructured data sources, such as text, images, and videos, to gain deeper understanding and uncover hidden patterns.
Examples: Natural language processing (NLP), sentiment analysis, image recognition.
6. Real-time Analytics:
Definition: Real-time analytics processes and analyzes data in near real-time, enabling organizations to respond quickly to changing conditions and make timely decisions.
Purpose: Real-time analytics provides instant insights into business operations, customer interactions, and market trends, enabling agile and adaptive decision-making.
Examples: Streaming analytics, event processing, real-time dashboards.
Conclusion:
Business Intelligence models provide a structured framework for organizations to analyze data, gain insights, and make informed decisions. By leveraging descriptive, diagnostic, predictive, prescriptive, cognitive, and real-time analytics, organizations can unlock the full potential of their data assets and gain a competitive edge in today’s dynamic business environment. Whether it’s understanding past performance, predicting future trends, or prescribing optimal strategies, BI models play a crucial role in driving business success through data-driven decision-making.